Towards robust, low power and adjustable accuracy Bayesian computers
- Share
- Share on Facebook
- Share on X
- Share on LinkedIn
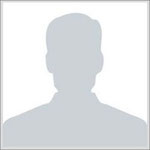
Stochastic computing, Sensor fusion, RNG,Bayesian inference, AI,Low power
Artificial intelligence (AI) is already revolutionizing our habits through technologies that allow the analysis, filtering and classification of a large amount of data that cannot be processed by conventional algorithms due to their complexity and incompleteness. Thus, there is now a strong demand for AI dedicated hardware while objects capable of capturing, collecting, processing and distributing information are more and more constrained in energy when the computing power required is constantly increasing. In this context, the Bayesian approach seems to be a particularly interesting way to carry out low cost and low power information fusion tasks.
By reducing multiplication to a single AND logic gate, stochastic arithmetic significantly reduces the size of operators (and therefore manufacturing costs) and their consumption at the expense of a lower computing speed, making it particularly suitable for Bayesian inference calculations. Initial results show the energy efficiency of this stochastic approach when the size of the problem to be treated is reduced and the accuracy required is low.
These results nevertheless raise two main bottlenecks when the size of the problem increases: the memory space needed to store probability distributions and the energy cost to generate random numbers.
This thesis aims to design Bayesian machine architectures that are efficient in low and high accuracy with a low energy consumption. To do so, it is necessary, on the one hand, to bypass the limits defined previously by rethinking the methods of storing distributions and generating random numbers necessary for stochastic calculation. And on the other hand, to exploit the specificities of these architectures, such as robustness allowing the increase of PVT margins and therefore the reduction of the circuit supply voltage, or the event-based nature of the Bayesian approach making an asynchronous implementation relevant.
By reducing multiplication to a single AND logic gate, stochastic arithmetic significantly reduces the size of operators (and therefore manufacturing costs) and their consumption at the expense of a lower computing speed, making it particularly suitable for Bayesian inference calculations. Initial results show the energy efficiency of this stochastic approach when the size of the problem to be treated is reduced and the accuracy required is low.
These results nevertheless raise two main bottlenecks when the size of the problem increases: the memory space needed to store probability distributions and the energy cost to generate random numbers.
This thesis aims to design Bayesian machine architectures that are efficient in low and high accuracy with a low energy consumption. To do so, it is necessary, on the one hand, to bypass the limits defined previously by rethinking the methods of storing distributions and generating random numbers necessary for stochastic calculation. And on the other hand, to exploit the specificities of these architectures, such as robustness allowing the increase of PVT margins and therefore the reduction of the circuit supply voltage, or the event-based nature of the Bayesian approach making an asynchronous implementation relevant.
Informations
Thesis director: Laurent FESQUET
Thesis supervisor: Karim CHERKAOUI (Hawai.Tech)
Thesis started on: Sept. 2019
Thesis defence: 12/10/2022
Doctoral school: EEATS
- Share
- Share on Facebook
- Share on X
- Share on LinkedIn